Fuel AI Success With the Right Data and the Right People
- Justin Ouimet
- Oct 28, 2024
- 3 min read
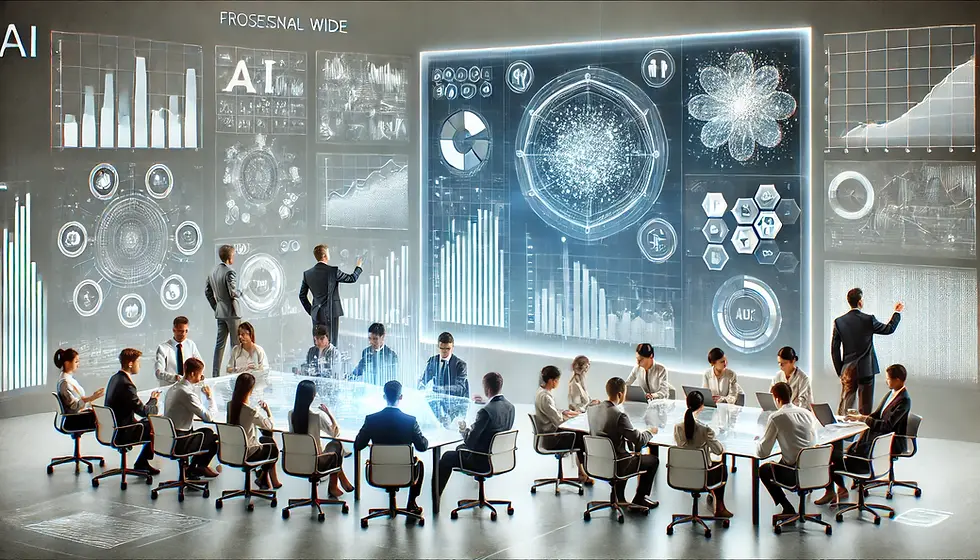
In today's AI-driven world, organizations are racing to build models that can deliver valuable insights, enhance operations, and drive competitive advantage. Yet, as highlighted by industry experts, the focus is often too heavily placed on technology and algorithms, sometimes at the expense of data quality and diverse, collaborative teams. This article dives into key points from a recent MIT Sloan Management Review webinar, sponsored by SAS, which discussed the role of high-quality data and multi-disciplinary teams in driving sustainable AI success.
Why Data Quality is Key to AI Success
Quality data underpins AI's ability to generate reliable results, yet achieving "right data" standards is more complex than it appears. Tom Redman, known as the "Data Doc," emphasizes that AI data quality is multi-dimensional, involving two primary components:
The Right Data – Data must be relevant to the AI model’s intended problem-solving scope.
Data Accuracy – Ensuring that the data used is accurate and free from biases.
With AI, ensuring the data is right for the model often involves carefully curated datasets tailored to specific use cases rather than simply leveraging large datasets that may not meet the model's requirements. This step is vital for AI's real-world applicability, whether in business analytics, finance, or personalized customer service.
The Role of Managers in Data Integrity
AI’s effectiveness is not solely in the hands of data scientists and modelers. Leadership has a critical role in setting the tone for data quality and holding responsibility for ensuring the right data is used. This responsibility involves clearly defining project goals, asking the right questions, and ensuring data relevance. Managers are also responsible for bridging the gap between data scientists and other team members, aligning goals and expectations across diverse disciplines.
As Redman noted, leaving the responsibility of data selection and model application entirely to the technical team can lead to misalignments, as data scientists and modelers may become too focused on refining their algorithms rather than assessing the data's strategic relevance. This disconnect is where leadership must step in to guide AI efforts toward achieving broader organizational goals.
Building a Diverse, Cross-Functional Team
A successful AI project isn’t just about having skilled data scientists; it requires a team with varied expertise. While statisticians and data scientists are integral to model building, experts in the business domain add value by ensuring that the model aligns with real-world use cases. Key skills that enhance an AI team’s success include:
Statistical Process Control – Enables teams to track data consistency over time.
Bayesian Methods – Allows integration of existing domain knowledge, enriching model accuracy.
Design of Experiments – Focuses on acquiring optimal data to answer specific questions, making the model as relevant and accurate as possible.
By combining expertise across these areas, organizations can maximize their model's accuracy and relevance while providing transparency and accountability.
Adapting to Limitations and Continuous Improvement
AI models trained on limited datasets can struggle when applied to new data under different circumstances, as they lack the "basis for inference" that allows them to generalize well. Redman and Roger Hoerl, a statistics professor, suggested that teams may consider developing a “statistical twin” to run parallel to complex AI models, allowing for transparency, interpretability, and error quantification. This twin can serve as a simplified counterpart, offering insights and cross-checks for more nuanced decision-making.
Additionally, maintaining ongoing monitoring processes—like statistical process control—is crucial in evaluating how AI models perform with new, real-time data and keeping them adaptable to shifting environments.
The Future: Smaller, Focused Language Models
With generative AI models and large language models (LLMs) becoming more popular, experts predict a shift toward smaller, focused models tailored for specific business applications. Rather than using broad internet datasets, these models could be trained on curated, industry-specific data, ensuring relevance and accuracy while maintaining privacy and quality. This move toward "small language models" represents a strategic focus on purpose-built, high-quality datasets that cater to specific organizational needs without the pitfalls of generalized data.
Building Trust in AI Through Transparency
The journey toward responsible, effective AI involves a combination of high-quality data, cross-functional collaboration, and executive oversight. As AI becomes increasingly central to business, organizations must prioritize transparency, accountability, and a deep understanding of data integrity. By focusing on these pillars, companies can foster trust in AI systems, enabling transformative benefits that are not only innovative but also sustainable.
Aligning Technology, Data, and Leadership for AI Success
As organizations continue investing in AI, it’s critical to balance cutting-edge algorithms with a commitment to data quality and diverse, collaborative teams. Leaders must step up to ensure their AI initiatives are grounded in relevance and ethical practices. By aligning technology, data integrity, and interdisciplinary expertise, organizations can drive lasting success with AI, setting themselves apart as leaders in the digital transformation journey.
#AILeadership #DataQuality #MachineLearning #AIInnovation #RightData #TechTrends #DigitalTransformation #AIModels #BusinessIntelligence #AIandData
Comments